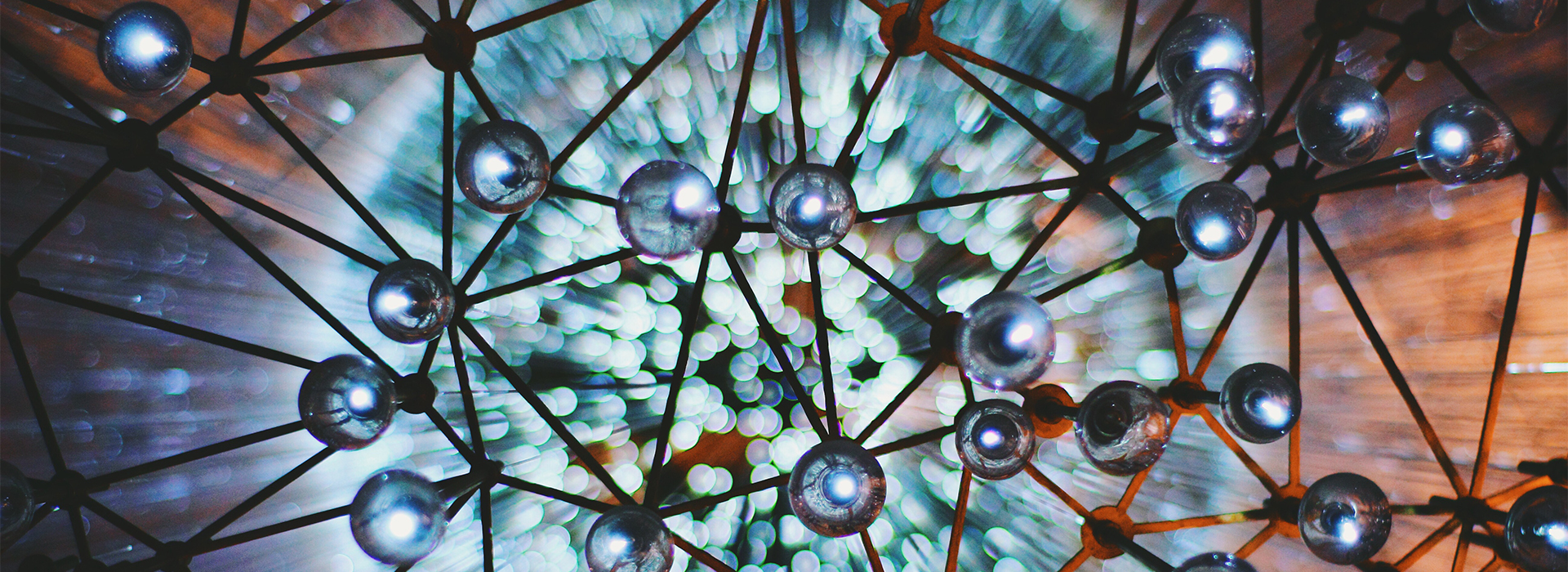
Research
-
A novel composite measure of risk-benefit for randomized clinical trials
A time-to-first endpoint (e.g., death or disease progression) is commonly analyzed but may not be adequate to summarize patient outcomes if a subsequent event contains important additional information. Settings of interest include those where both survival and a surrogate outcome that indicates a beneficial outcome are both observed, so that the usual time-to-first endpoint of death or surrogate event is nonsensical. We developed a new two sample test for bivariate, interval-censored time-to-event data, where one endpoint is a surrogate for the second, less frequently observed endpoint of true interest (https://pubmed.ncbi.nlm.nih.gov/27059817/). This test examines whether patient groups have equal clinical severity. We apply this rank statistic to summarize clinical severity for subjects in a trial comparing the efficacy of two interventions for tuberculosis (TB) outcomes. The proposed test statistic compares clinical severity using a composite score and a log-rank type statistic that utilized the bivariate survival outcome for the two event times (time to TB conversion and death). This work has connections with other prioritized outcome approaches, such as the win ratio; however, in contrast to the win ratio, the developed test statistic makes use of the survival distribution of all outcomes. A direct comparison of these approaches is considered in a recent article in Clinical Trials, whose focus was on methods to summarize the benefit-risk ratio (https://pubmed.ncbi.nlm.nih.gov/30021496/). The clinical severity approach has also been applied in a randomized clinical trial examining the efficacy of convalescent plasma for hospitalized COVID19 patients (ClinicalTrials.gov Identifier NCT04397757).
-
Methods to improve hazard ratio inference clinical trials with time-to-event endpoints and limited sample size
Many early phase clinical trials involve time-to-event endpoints with limited sample size. We considered a refined generalized log-rank statistic that used the binomial distribution of the binary outcomes at each failure time to derive the underlying distribution of the test statistic. For both the stratified and unstratified Cox model (https://www.tandfonline.com/doi/abs/10.1080/19466315.2017.1369899; https://pubmed.ncbi.nlm.nih.gov/30706642/), we were able to demonstrate efficiency gains over the usual log-rank test for the two-group treatment comparison. Further, for settings of cross-over trials with event-time outcomes, such as bleeding time for studies comparing anticoagulants or stopping times from stress tests for trials of drugs managing cardiac symptoms, we also considered how to incorporate baseline outcome information into the analysis of treatment effect to increase efficiency (https://pubmed.ncbi.nlm.nih.gov/29888552/).
Diagnosing Fraudulent Baseline Data in Clinical Trials. https://pubmed.ncbi.nlm.nih.gov/32998158/
-
Estimation Methods to handle measurement error
Data in large observational studies and in studies reliant on electronic health records can be prone to measurement error. In recent work, we have studied estimators that allow for valid inference in settings with an error-prone failure time outcome, possibly also with error-prone exposures. In settings where a validation substudy, on which the true data have been observed, is available, raking estimators are a robust and efficient option. Raking estimators treat the error-prone observations as auxiliary variables, which are used to improve the efficiency of the usual inverse probability weighted (Horvitz-Thompson) estimator. Raking estimators, in contrast to regression calibration, have the benefit of not having to directly model the measurement error, which can be challenging in the context of EHR. In recent work, we demonstrated that the design-based raking estimator maintained advantages in terms of mean-squared error over common parametric and semi-parametric estimators, including standard multiple imputation, even in settings of mild model misspecification (arXiv:1910.01162[stat.ME]). We have examined the performance of raking estimators in comparison with regression calibration and more naïve estimators (https://pubmed.ncbi.nlm.nih.gov/33140432/) and have examined how to improve the efficiency of the raking estimator with the choice of the raking variable and study design (https://pubmed.ncbi.nlm.nih.gov/33709462/).
-
Two-phase analysis and study design for survival models with error-prone exposures
Audits and validation studies data to understand the error structure are vital for these methods, and we have advocated for the collection of such data to improve inference in these settings (https://pubmed.ncbi.nlm.nih.gov/27365013/; https://pubmed.ncbi.nlm.nih.gov/22848072/; https://www.degruyter.com/document/doi/10.1515/scid-2019-0015/html) . We have adapted the mean score estimator to the discrete survival setting and developed a multi-phase optimal design for two phase discrete survival data, which also was shown to improve efficiency in the continuous survival time setting (https://pubmed.ncbi.nlm.nih.gov/33327876/). This is an important contribution to two-phase study designs, as it allows for practical and cost-effective validation designs for the general survival setting, as well as a robust estimation method that avoids untestable assumptions about the measurement error structure. In this work we also explored adaptive two-wave sampling.
-
Statistical Guidance for handling measurement error
Together with members of the STRATOS Measurement Error and Misclassification Topic Group (TG4) ( http://www.stratostg4.statistik.uni-muenchen.de), we conducted a literature review of current practice on handling measurement error in areas of epidemiology focused on exposures known to be error-prone and found that most researchers either ignored or inadequately handled measurement error in their study analyses (https://pubmed.ncbi.nlm.nih.gov/30316629/ ). TG4 authored two guidance papers methods to handle measurement error and misclassification in study design and data analyses. (https://pubmed.ncbi.nlm.nih.gov/32246539/; https://pubmed.ncbi.nlm.nih.gov/32246531/) Dr. Shaw has also written a chapter describing the popular method Regression Calibration for a forthcoming book on measurement error (https://www.routledge.com/Handbook-of-Measurement-Error/Yi-Delaigle-Gustafson/p/book/9781138106406).
-
Impact of Regression to the Mean on the Synthetic Control Method : Bias and Sensitivity Analysis
-
Nutritional and Physical Activity Epidemiology
Dietary intake and physical activity exposures are highly variable and generally measured with error. For some dietary intakes, recovery biomarkers are available on a subset of participants, which allows for calibration of self-reported data on the larger cohort in order to adjust for the error in the self-reported exposure. These techniques are applied using data from the Study of Latinos Nutrition & Physical Activity Assessment Study (SOLNAS) within the large multi-site Hispanic Community Health Study/ Study of Latinos, a biomarker study that has provided insights into the measurement error structure in the main study instruments for several dietary nutrients (https://pubmed.ncbi.nlm.nih.gov/25995289/; https://pubmed.ncbi.nlm.nih.gov/28205551/; https://pubmed.ncbi.nlm.nih.gov/27339078/) and physical activity (https://pubmed.ncbi.nlm.nih.gov/30177242/). SOLNAS has provided the first look at measurement error of these common instruments in a largely understudied population of Hispanics and studies are now under way to use the measurement error-corrected exposures to study the association between diabetes and cardiovascular outcomes. Dr. Shaw collaborated on with WHI investigators to develop calibration equations for energy and protein in the WHI cohort (https://pubmed.ncbi.nlm.nih.gov/18344516/ ) and examined measurement error-corrected diet-disease associations for several cancer outcomes (https://pubmed.ncbi.nlm.nih.gov/19258487/). She has also collaborated on the design and analysis of a human feeding study that studied and has found promising results for novel new nutritional biomarkers based on stable isotope ratios for nitrogen and carbon (https://pubmed.ncbi.nlm.nih.gov/31515553/; https://pubmed.ncbi.nlm.nih.gov/33676366/).
Early phase studies of safety and efficacy for Chimeric Antigen Receptor (CAR) T-cell Therapy. Dr. Shaw has had a lead statistical role in early phase immunotherapy studies for both cancer and HIV, including the Penn clinical trials of CTL019 for the treatment of relapsed and refractory adult and pediatric acute lymphoblastic leukemia (ALL). CTL019 went on to become the first FDA-approved gene therapy available in the United States. She led the statistical analysis for a landmark publication summarizing the treatment success for ALL in the New England Journal of Medicine (https://pubmed.ncbi.nlm.nih.gov/25317870/). She also led the development of prediction models to determine which subjects were at higher risk for the life-threatening adverse event of cytokine release syndrome (https://pubmed.ncbi.nlm.nih.gov/27076371/) and which were at higher risk for neurotoxicity (https://pubmed.ncbi.nlm.nih.gov/30178481/), as well as developed a model to better understand the distinguishing features of CRS versus sepsis in a pediatric population (https://pubmed.ncbi.nlm.nih.gov/33095872/). She further helped evaluate the dose that best balanced safety and efficacy of CTL019 in adults with ALL (https://pubmed.ncbi.nlm.nih.gov/31815579/). In this analysis, one question of interest was how the survival experience differed between individuals who did or did not receive a stem cell transplant (SCT) after going into remission after treatment with CTL019.